What is hybrid quantum computing? Let’s explore the link between quantum and classical computers!
Since the idea of the quantum computer was born in the 1980s, it has drawn great attention due to its promise to solve classically intractable problems. Particularly in the last decade, there has been substantial academic progress, and the field has witnessed an outstanding series of results delivered by the quantum information science community to bring this idea to reality. Today, we are standing at the forefront of this development, looking at a range of big companies and startups that are paving the way out of the lab towards the realization of useful quantum computing systems for real-life applications.But why are quantum computers interesting, and what can they accomplish? How might a quantum computing system appear to be in production? Read on to find out the answers.
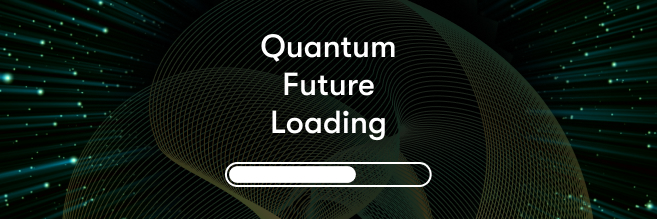
FAQ – Frequently Asked Questions
Q1: How does hybrid quantum computing work?
The core idea behind hybrid quantum computing is that quantum computers work as co-processors alongside classical systems, where both carry out parts of the computational workload. Alternative interpretations of the term hybrid, however, may vary based on contextual distinctions, including different system architectures and types of tasks taken over by the involved compute resources. For example, quantum computers always rely on classical computers to control the physical operations of the quantum device or enable remote access. Some architectural approaches also involve high-performance computing (HPC) systems to accelerate helper tasks such as compilation, error mitigation, and data preprocessing for quantum computers.
Q2: What are the benefits of hybrid quantum computing?
Quantum computers will only execute certain parts of a program. Hybrid algorithms facilitate the division of computational tasks between quantum and classical computing resources, capitalizing on the strengths of each. While numerous quantum algorithms require error correction and extensive physical resources, recently developed hybrid quantum algorithms, such as variational quantum algorithms, ensure that certain tasks can be reliably executed with current quantum technology.
Q3: What are the challenges of implementing hybrid quantum-classical algorithms?
Implementing hybrid quantum-classical algorithms poses several challenges regarding system architecture, hardware and software compatibility, resource management, and more. Overcoming these challenges requires innovative approaches from hardware design to software engineering and algorithm development. Collaboration among academia, industry, and government agencies will play a pivotal role in advancing hybrid quantum-classical computing technology.
Why are quantum computers interesting, and what can they do (better)?
First, what is a quantum computer?
Quantum computers promise supreme computational capabilities through their natural ability to process quantum information. The basic unit of quantum information is called a quantum bit (or qubit), the counterpart of a classical bit. Quantum bits are physically encoded in a two-levelled quantum system, such as the polarization of single photons or the spin of electrons. Using quantum systems for information processing allows for exploiting non-classical phenomena such as superposition, entanglement, and interference to represent data and carry out computations.
A multitude of small- to medium-scale realizations of quantum processors with a few hundred up to a thousand qubits and based on a variety of different hardware – ranging from atomic and ionic systems, superconducting qubits, to solid-state and optical platforms – already exist [1, 2]. Furthermore, experimental realizations with superconducting qubits as well as with optical setups have demonstrated superior performance compared to leading supercomputers in specific computational tasks, i.e., boson sampling [3, 4, 5]. Although the physical number of qubits has increased significantly in recent years, today’s quantum hardware still suffers from limited scaling potential, qubit coherence and connectivity, and other device imperfections. These imperfections introduce noise to the computation that ultimately destroys the valuable non-classical properties, thereby posing physical limits to the quantum computations that can be executed.
What can quantum computers do (better)?
Quantum systems offer a new computational paradigm, fundamentally different from classical computation. Theory states that for certain problems, quantum algorithms scale more efficiently with larger problem sizes than the best-known classical algorithms [1]. Therefore, quantum computers are expected to provide a computational advantage over classical computers when solving specific tasks. Well-known examples include search-related tasks, factorization of large numbers, and the simulation of molecular dynamics.
This extends further to a broad range of possible applications, including the simulation of complex engineering processes and chemical systems for the development of new materials and drug discovery, optimization of routing and supply chains in the production and logistics domain, as well as insurance risk assessment [6].
However, large-scale, fault-tolerant quantum computers that could revolutionize industries from automotive, manufacturing, chemical, and pharmaceutical production to insurance and finance remain theoretical for the time being. Today’s quantum computers are still in an early stage of development and have not yet demonstrated an advantage in solving any real-world business or industry problems. Currently, available quantum hardware is error-prone and intermediate in scale, with up to a thousand qubits, coining the term noisy-intermediate-scale-quantum (NISQ) era. One of the challenges that remains in the near future is overcoming some of their current limitations.
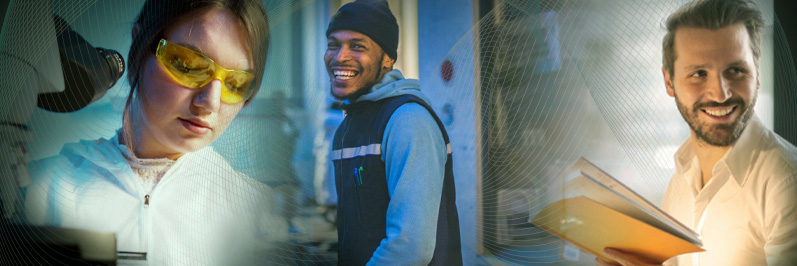
Hybrid quantum computing approaches
Introducing quantum for classical systems
Hybrid quantum-classical computing systems present an exciting possibility. The idea is that quantum computers work as co-processors alongside classical systems. Standard classical computers are already required to control the physical operation of quantum gates or enable remote access. In that sense, a quantum processor will always work in tandem with a classical computer, although the reverse is not true. Other architectural visions rely on high-performance computing (HPC) systems to speed up classical helper tasks such as compilation, error mitigation, error correction, preprocessing the input data for the quantum computer, as well as post-processing and analysis [7, 8]. Academia and industry are also focusing on the development of hybrid algorithms that split a computational workload between quantum and classical computing resources, aiming to use each resource for what it does best [9, 10].
Based on recent developments of NISQ devices, it is envisioned that a first quantum advantage for an industrially relevant problem will be achieved through a hybrid quantum-classical combination of traditional HPC systems with a quantum computer as an accelerator [7]. What the eventual architecture and design of such a hybrid system could look like can only be sketched today and is an extensively studied research topic. Supercomputing centers and research groups around the world are investigating this HPC-integrated approach to quantum computing. Prominent examples are Forschungszentrum Jülich, Barcelona Supercomputing Center, CINECA, Oak Ridge National Laboratory, Pawsey Supercomputing Centre, and many more.
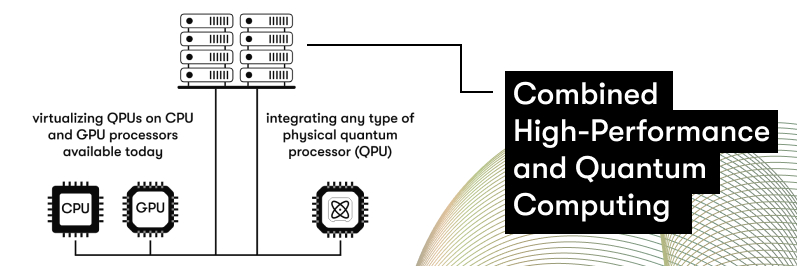
Quantum-Classical Synergy in Action
Many proposals for quantum algorithms require error correction and demand more resources than today’s NISQ technology can provide, limiting their usefulness in the near future. However, variational quantum algorithms (VQAs) are a class of hybrid quantum-classical algorithms that show great potential and are particularly suited for use with NISQ devices [9]. They are built on a quantum-classical loop, where the quantum part consists of parameterized quantum gates whose parameters are trained and updated using a classical optimization algorithm. As the algorithm iterates, tasks are assigned to the quantum processor and the classical computer. VQAs have been demonstrated in various proof-of-concept implementations, e.g., with photonic [12] and ionic systems [13]. They also provide a realistic approach to leveraging the full capabilities of near-term hybrid systems, as they are commonly used in conventional HPC applications such as chemistry simulations, machine learning, and optimization problems [9, 11].
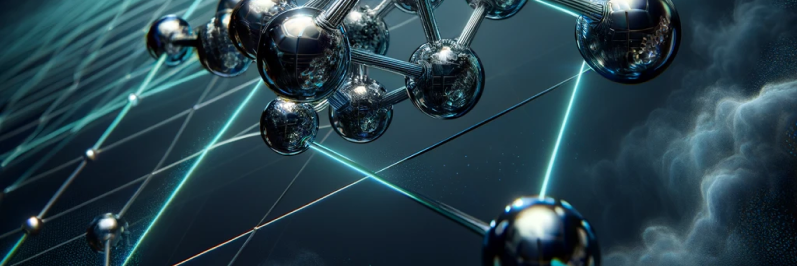
Real-World Applications Across Industries
Quantum computing finds applications across industries. Below, you will find some examples of recent collaborations addressing various use cases:
Quantum Mobility Quest by Airbus and BMW Group
To cite a recent announcement, Airbus and BMW Group have partnered to launch the Quantum Mobility Quest, an innovative initiative aimed at revolutionizing the transportation sector through quantum computing. This global challenge focuses on harnessing quantum technologies to address key issues in the aviation and automotive industries. Participants are invited to tackle one of five problem statements, the winning team is to be announced by the end of the year.
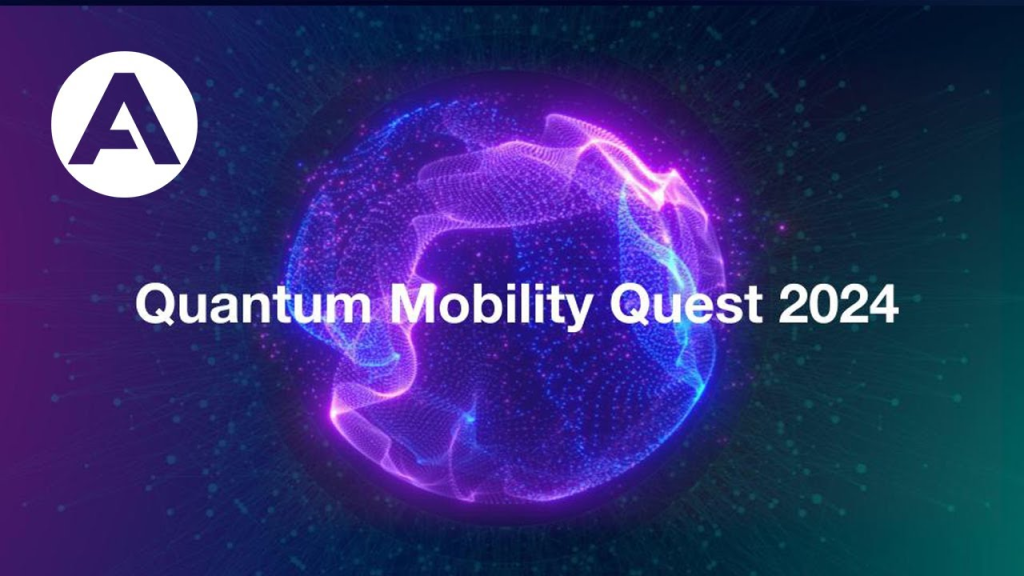
Airbus:
In partnership with the BMW Group and Quantinuum, Airbus is working on simulating the oxygen reduction reaction on the surface of a platinum-based catalyst using quantum computing. This reaction is critical in the process that converts hydrogen and oxygen into water and electricity in a fuel cell. Understanding this process is expected to lead to the development of alternative materials that may improve the performance and reduce the production costs of fuel cells, crucial for sustainable hydrogen-powered alternatives in aviation.
Thales Group:
Thales Group, in collaboration with Terra Quantum, has applied hybrid quantum computing to optimize satellite mission planning. They’ve demonstrated significant potential for improving satellite operational utility using a hybridized quantum-enhanced reinforcement learning approach. According to The Quantum Insider, Terra Quantum and Thales Group estimate their model could generate an estimated EUR 22,500 of value creation potential per satellite per day.
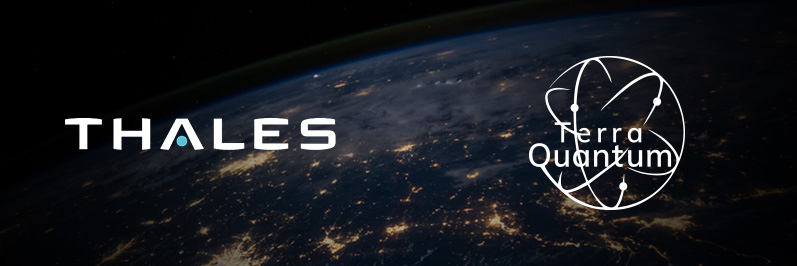
Outlook and further information
Developments in (hybrid) quantum computing are rapidly emerging, driven by academic research and industry advancements. While the potential for transformative applications is immense, it is crucial to approach the technology with a balanced perspective. We believe that access to quantum computing resources is crucial for forming realistic expectations in targeted industries. That’s why QMware is building a cloud platform that supports the heterogeneous use of emulated and physical QPUs from across the technology spectrum, enabling our clients to leverage quantum acceleration for their workflows and achieve production readiness.
We understand that realizing the full promise of quantum computing requires collective effort. That’s why QMware is part of projects such as Quantum Ready and Quantum Connect in Austria, as well as the German SeQuenC initiative led by IONOS and supported by PlanQK, Fraunhofer Fokus, and the University of Stuttgart. The goal is to provide access to classical and quantum computing resources for SMEs and industries alike.
For a more detailed understanding of the current status and future outlook in this field, we recommend the latest edition of the State of Quantum Report 2024, published by Lakestar in collaboration with IQM, Open Ocean, and The Quantum Insider.
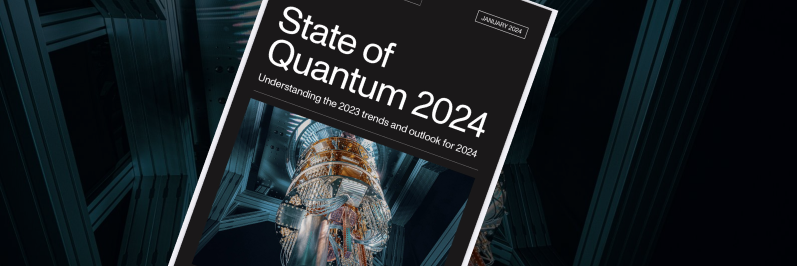
Curious about what hybrid quantum computing has in store for your business? Discover how QMware’s solutions can redefine your computing capabilities!
References
[1] Nielsen, Michael A., and Isaac L. Chuang. 2012. “Quantum Computation and Quantum Information.” Cambridge University Press. https://doi.org/10.1017/cbo9780511976667.
[2] Castelvecchi, Davide. 2023. “IBM Releases First-Ever 1,000-Qubit Quantum Chip.” Nature. Springer Science and Business Media LLC. https://doi.org/10.1038/d41586-023-03854-1.
[3] Arute, Frank, Kunal Arya, Ryan Babbush, Dave Bacon, Joseph C. Bardin, Rami Barends, Rupak Biswas, et al. 2019. “Quantum Supremacy Using a Programmable Superconducting Processor.” Nature. Springer Science and Business Media LLC. https://doi.org/10.1038/s41586-019-1666-5.
[4] Zhong, Han-Sen, Hui Wang, Yu-Hao Deng, Ming-Cheng Chen, Li-Chao Peng, Yi-Han Luo, Jian Qin, et al. 2020. “Quantum Computational Advantage Using Photons.” Science. American Association for the Advancement of Science (AAAS). https://doi.org/10.1126/science.abe8770.
[5] Madsen, Lars S., Fabian Laudenbach, Mohsen Falamarzi. Askarani, Fabien Rortais, Trevor Vincent, Jacob F. F. Bulmer, Filippo M. Miatto, et al. 2022. “Quantum Computational Advantage with a Programmable Photonic Processor.” Nature. Springer Science and Business Media LLC. https://doi.org/10.1038/s41586-022-04725-x.
[6] Andreas Bayerstadler, Guillaume Becquin, Julia Binder, Thierry Botter, Hans Ehm, Thomas Ehmer, et al. 2021. “Industry Quantum Computing Applications.” EPJ Quantum Technology. Springer Science and Business Media LLC. https://doi.org/10.1140/epjqt/s40507-021-00114-x.
[7] Bertels, K., A. Sarkar, A. Krol, R. Budhrani, J. Samadi, E. Geoffroy, J. Matos, R. Abreu, G. Gielen, and I. Ashraf. 2021. “Quantum Accelerator Stack: A Research Roadmap.” arXiv. https://doi.org/10.48550/ARXIV.2102.02035.
[8] Perelshtein, Michael, Asel Sagingalieva, Karan Pinto, Vishal Shete, Alexey Pakhomchik, Artem Melnikov, Florian Neukart, Georg Gesek, Alexey Melnikov, and Valerii Vinokur. 2022. “Practical Application-Specific Advantage through Hybrid Quantum Computing.” arXiv. https://doi.org/10.48550/ARXIV.2205.04858.
[9] Cerezo, M., Andrew Arrasmith, Ryan Babbush, Simon C. Benjamin, Suguru Endo, Keisuke Fujii, Jarrod R. McClean, et al. 2021. “Variational Quantum Algorithms.” Nature Reviews Physics. Springer Science and Business Media LLC. https://doi.org/10.1038/s42254-021-00348-9.
[10] Weigold, Manuela, Johanna Barzen, Frank Leymann, and Daniel Vietz. 2021. “Patterns for Hybrid Quantum Algorithms.” Service-Oriented Computing. Springer International Publishing. https://doi.org/10.1007/978-3-030-87568-8_2.
[11] Cranganore, Sandeep Suresh, Vincenzo De Maio, Ivona Brandic, Tu Mai Anh Do, and Ewa Deelman. 2022. “Molecular Dynamics Workflow Decomposition for Hybrid Classic/Quantum Systems.” 2022 IEEE 18th International Conference on E-Science (e-Science). IEEE. https://doi.org/10.1109/escience55777.2022.00048.
[12] Peruzzo, Alberto, Jarrod McClean, Peter Shadbolt, Man-Hong Yung, Xiao-Qi Zhou, Peter J. Love, Alán Aspuru-Guzik, and Jeremy L. O’Brien. 2014. “A Variational Eigenvalue Solver on a Photonic Quantum Processor.” Nature Communications. Springer Science and Business Media LLC. https://doi.org/10.1038/ncomms5213.
[13] Hempel, Cornelius, Christine Maier, Jonathan Romero, Jarrod McClean, Thomas Monz, Heng Shen, Petar Jurcevic, et al. 2018. “Quantum Chemistry Calculations on a Trapped-Ion Quantum Simulator.” Physical Review X. American Physical Society (APS). https://doi.org/10.1103/physrevx.8.031022.